At AI Infrastructure Field Day 2 (AIIFD2), Nutanix presented Nutanix Enterprise AI (NAI) platform, a solution designed to make enterprise-grade GenAI deployments simpler, more secure, and operationally viable. The intent is laudable, but does it deliver? And what issues are organizations facing when it comes to deploying GenAI solutions?
The Challenge: AI at Enterprise Scale
Enterprises face several hurdles when adopting GenAI. Infrastructure complexity leads the list. Teams often struggle with basic questions: what hardware to choose, how to configure the environment, or how to scale from a prototype to production. A project that starts in a lab can quickly become business-critical, but without the architecture to support that growth. Furthermore, initial design choices / sunken costs can later negatively impact the outcomes.
Security and compliance add to the challenge. It’s common to pull models from public sources without knowing their origin or trustworthiness. There’s often no clarity on whether these models are secure, auditable, or compliant. Without proper governance, model deployments can lead the organization towards typical shadow IT challenges, exposing the business to risk due to lack of control and visibility.
What the Ideal Platform Looks Like
To bring GenAI to the enterprise in a sustainable way, the right platform needs to address a few key requirements:
- Secure and validated models, to ensure trust and reduce risk
- Scalable and portable architecture, leveraging containers and Kubernetes for seamless mobility across environments, either on-prem or in public clouds
- Built-in governance, including observability, auditability, and role-based access controls
- Operational efficiency, with curated model libraries and ready-to-deploy templates
- On-prem and cloud parity, so AI workloads can evolve without friction or lock-in
Enter Nutanix Enterprise AI (NAI)
Nutanix Enterprise AI is a turnkey solution built with these principles in mind. Based on Kubernetes and fully infrastructure-agnostic, NAI runs on any CNCF-certified Kubernetes distribution such as GKE, EKS, AKS, or on-prem clusters.
It includes a curated library of pre-validated LLMs, with models from Hugging Face, NVIDIA NIM, and NVIDIA NeMo. Organizations can also bring their own custom models, giving them flexibility to address specific use cases.
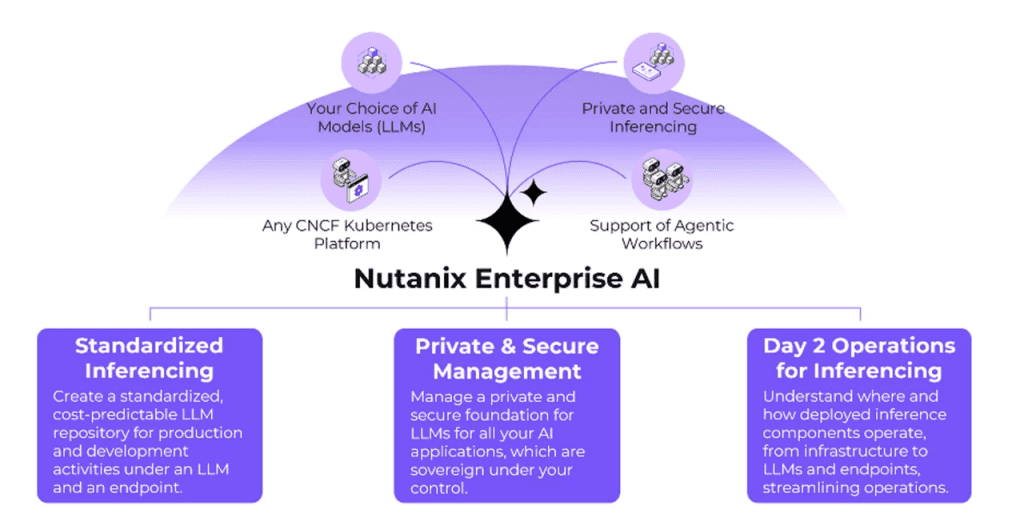
Deployment is straightforward. Users pick a model and deploy it using pre-defined templates that recommend optimal CPU, RAM, GPU, and storage settings. For cloud deployments, NAI helps identify the best GPU-optimized instances based on the chosen provider.
From a security and operations perspective, NAI provides secure endpoints for accessing models, along with a central management interface offering detailed observability. Teams get visibility into API calls, endpoint status, GPU consumption, and cluster health. Role-based access controls help maintain policy-driven, secure usage across teams.
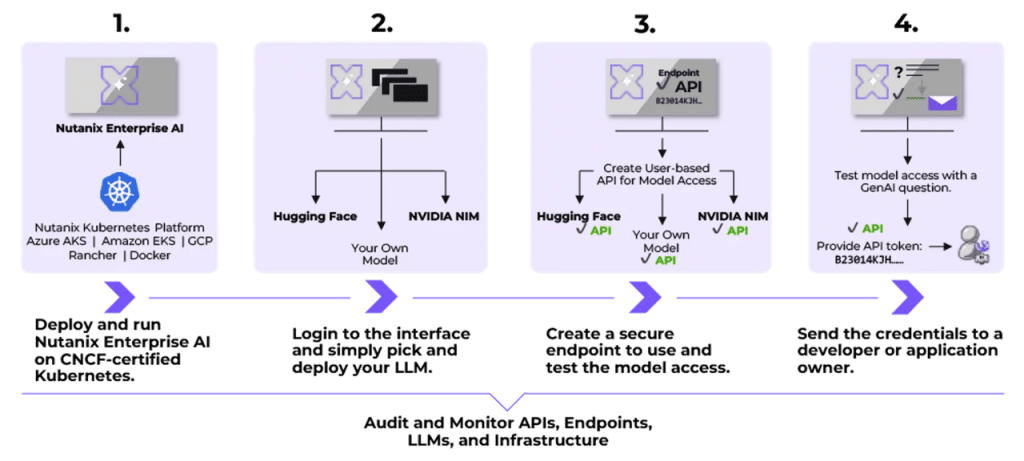
The Osmium Perspective
With NAI, Nutanix is offering a practical entry point for organizations exploring GenAI. Its flexibility to run anywhere means teams can start in the cloud and move on-prem as needs evolve. The platform removes much of the complexity from AI infrastructure and makes model management simple & straightforward.
At the same time, it meets the requirements of organizations with mature governance/compliance frameworks in place. With its approach, it allows those organizations to deploy LLMs in a controlled manner, be it from a security or access management standpoint.
Lastly, the solution is platform agnostic and does not require underlying Nutanix infrastructure to operate.
Lastly, Osmium Data Group covered Nutanix AI in episode 6 of the Osmium Update:
Additional Content
To find out more, watch the AIIFD2 Nutanix sessions below: